2 Philip Morris International R&D;, Philip Morris Products S.A., Neuchâtel, Switzerland,
3 Philip Morris International R&D;, Philip Morris Research Laboratories GmbH, Cologne, Germany
Abstract
Motivation:Atherosclerosis is a complex multi-pathway inflammatory disease where accumulation of oxidatively modified lipids and leukocytes in the arterial intima leads to plaque formation over time. Translating Apoe-/- mouse results to the clinical setting is complicated by uncertainty around (a) mechanisms underlying disease etiology, (b) relative importance of these mechanisms as drivers of progression, and (c) how these roles change in response to perturbation by therapeutic intervention or lifestyle changes.
Results: We describe a large-scale mechanistic, mathematical model of atherosclerosis in the Apoe-/- mouse and its validation with in vivo Apoe -/- data. Major physiological components include cholesterol/macrophage trafficking, inflammation, endothelial function, oxidative stress, and thrombosis. Heterogeneity in disease progression, observed despite genetic uniformity and experimentally controlled conditions, was captured through “virtual mice”. This model may be used to optimize in vivo experiments and paves the way for a similar modeling approach for human disease.
Availability: The model is available by remote desktop client at Apoe.entelos.com.
Citation: Chan JR, Vuillaume G, Bever C, Lebrun S, Lietz M, Steffen Y, et al. The Apoe-/- Mouse PhysioLab® Platform: A Validated Physiologically-based Mathematical Model of Atherosclerotic Plaque Progression in the Apoe-/- Mouse. Biodiscovery 2012; 3: 2; DOI: 10.7750/BioDiscovery.2012.3.2
Copyright: © 2012 Chan et al. This is an open-access article distributed under the terms of the Creative Commons Attribution License, which permits unrestricted use, provided the original authors and source are credited.
Received: August 29, 2012; Accepted: September 24, 2012; Available online /Published: September 30, 2012
Keywords: atherosclerosis, Apoe-/- mouse, mathematical model, cigarette smoke
Abbreviations: checkpoint: mitotic spindle assembly checkpoint
*Corresponding Authors: Jason R Chan, email: chan@entelos.com Julia Hoeng, e-mail: julia.hoeng@pmi.com
# These authors contributed equally to this work
Conflict of Interests: The research described in this article was supported by Philip Morris International in a collaborative project with Entelos Holding Corporation. The PhysioLab® Platform is a licensed software developed by Entelos. The model described in this paper is accessible by remote desktop client at Apoe.entelos.com. Please contact support@entelos.com to obtain a user name and password.
Introduction
Atherosclerosis is an inflammatory disease characterized by the accumulation of lipoproteins and leukocytes as plaques in the arterial intima. Uncontrolled, it can lead to coronary heart disease (CHD), and underlying clinical events such as heart attack or angina. CHD caused approximately 1 of every 6 deaths in the United States in 2006 [1]. Development of CHD is accelerated by a variety of risk factors, including male gender, smoking,
dyslipidemia, elevated blood pressure, physical inactivity, obesity, and diabetes. Therapeutics targeted at reducing CHD face a difficult pre-clinical hurdle due to a dearth of appropriate animal models that capture the complexity of the human disease, which generally takes more than 50 years to result in a clinically apparent event. In addition, therapies for treating known risk factors (e.g., diabetes) have led to unexpected increases in CHD events outside of the clinical trial setting [2].
Genetically manipulated mice have become the predominant pre-clinical model for studying experimental atherosclerosis due to the ability to control confounding genetic variables, cost-effectiveness in generating large numbers of replicates, and the rapid development of a relatively complex disease compared to more-traditional rabbit, pig, and primate models. In particular, the apolipoprotein E knockout (Apoe-/-) mouse is the most widely used pre-clinical model of atherosclerosis [3]. As apo E is essential for the clearance of cholesterol from the circulation in mice, Apoe-/- mice have exceptionally high levels of serum cholesterol compared to wild-type, resulting in the development of lipid-containing lesions throughout the vascular tree. These lesions contain all of the cell types and features of human atherosclerotic plaques: cholesterol-engorged macrophages (foam cells), cholesterol clefts, acellular areas, fibrous caps, and calcification [4]. However, fundamental species differences remain, including (but not limited to) the fact that the lesions in the mouse do not result in clinical events as seen in humans. This fundamental difference adds a layer of uncertainty to the evaluation of therapeutic interventions or traditional risk factors in the mouse to predictions of human therapeutic responses.
Here we describe a mechanistic in silico model of atherosclerosis in the Apoe-/- mouse and its validation against laboratory data. Construction of the Apoe-/- PhysioLab platform utilized data from hundreds of scientific publications to represent plaque progression as a function of the physical size, geometry and composition of the arteries in which atherosclerosis forms. The plaque progression includes interaction of the endothelial layer with platelets, modification of circulating cholesterol particles and their accumulation in the arterial wall, activity of inflammatory cells, and the effects of common environmental factors (e.g., diet and exposure to cigarette smoke) as well as therapeutic interventions (e.g., ezetimibe) in the mouse. Due to its size, a full mathematical description of the entire platform is not reasonable within the body of this manuscript. However, illustrations of our modelling approach, the equations, assumptions, and data sources for submodules are summarized.
Materials and Methods
Model design
A top-down, outcomes-focused approach was used to develop the Apoe-/- PhysioLab platform. This staged and iterative process included four phases: (a) design, (b) architecture, (c) internal validation, and (d) external validation. The model scope was defined in the design phase to include (a) identification of system-level outputs (e.g., plaque progression) that describe the disease state, (b) biological components, functions, and interactions (e.g., macrophage recruitment, lipid modification and retention, thrombosis, etc.) needed to give rise to the system-level outputs, (c) the system-level behaviors (e.g., response to diets and/or therapeutic intervention) against which the simulation results are compared in order to validate virtual mice, which are unique parameterizations of the model that are consistent with these behaviors. Major biological components were selected based on demonstrated importance in disease. For example, the inclusion of macrophages is supported by many reports illustrating their early accumulation in the arterial intima and uncontrolled uptake of cholesterol resulting in a conversion to foam cells [5, 6]. The model scope is summarized in Table 1 and was based on a thorough review of the public literature. To provide a more detailed overview of the biology represented in the model, we describe the main model components, including their functional activities, modes of interaction and a selection of pertinent references. The complete set of references used in building and validating the model are documented within the model itself.

Cholesterol trafficking/Macrophage recruitment
Atherosclerotic plaque progression and regression are hypothesized to be primarily driven by the balance between cholesterol retention and efflux from the vessel [7], which is dependent on both the retention of circulating atherogenic particles (predominantly low density lipoprotein (LDL) in humans and very low density lipoprotein (VLDL)/intermediate density lipoprotein (IDL) in Apoe-/- mice) within the plaque and cholesterol efflux from the plaque to apo A-I particles [7, 8]. Within the arterial wall, retained cholesterol is taken up by monocyte derived macrophages recruited to the plaque by inflammatory mediators. As their lipid content increases faster than they can offload, they increase in size and develop intracellular vesicles containing the excess lipid. These foam cells are less mobile and contribute cholesterol to the lipid core of atherosclerotic lesion if they die prior to migrating from the plaque. In the model, all cells and processes involved in plaque growth and development are modulated by plaque geometry and inflammatory mediators. The model captures the recruitment of macrophages from circulation and their differentiation into macrophages and foam cells. Migration of macrophages from the plaque to the lymphatic system is also represented in the model, resulting in additional lipid efflux from the plaque, though this process is thought to be limited in the Apoe-/- mouse [9]. Data from histological samples on the plaque distribution and number of macrophages and foam cells were used to define the size of vascular cells and the geometry and rate of plaque progression (See Supplemental Table 1) in the model.
Inflammation
Endothelial cells (EC), smooth muscle cells (SMC), T-cell and monocyte derived cells in the plaque can be activated to produce mediators (e.g., cytokines, chemokines and growth factors). The model integrates the overall and continuously changing pro-inflammatory effects into a term that represents the magnitude of inflammation in the plaque, which is then used to regulate processes such as recruitment, activation, proliferation and death of macrophages, T-cells, SMCs and ECs and modification/retention of VLDL/IDL particles in the plaque.
Endothelial cell function
ECs are affected both by circulating mediators and the vascular wall that they overlie. Hypercholesterolemia can have direct effects leading to EC dysfunction, including impairment of nitric oxide (NO) and reactive oxygen species (ROS) production, which are hypothesized to increase the rate of plaque progression [10]. In atherosclerosis, dysfunctional ECs could be replaced by replication of local cells and by recruitment of endothelial progenitor cells (EPCs) from the bone marrow [11, 12]. The model captures the lifecycle of EPCs, ECs and production of key mediators such as NO and their effects on the other vascular cells.
Oxidative stress
Oxidative stress is associated with accelerated plaque progression and increased CHD risk [13]. ROS are mainly produced by monocyte derived cells, smooth muscle and endothelial cells in the arterial wall in response to hypercholesterolemia and inflammatory mediators. Their effects are amplified when production of atheroprotective endothelial nitric oxide (NO) is reduced by EC dysfunction. Both increased ROS and reduced endothelial NO [14-19] have been implicated in accelerating plaque progression in Apoe-/- mice and are represented in the model.
Thrombosis
Thrombosis is known to play an important role in atherogenesis [20]. Its representation in the Apoe-/- PhysioLab platform focuses on platelet activation, adhesion and aggregation to activated endothelium with release of inflammatory mediators which accelerate atherosclerotic plaque progression.
Model architecture
The mathematical relationships and interactions between the biological components of the mechanistic model of atherosclerosis were visually arranged and integrated together using algebraic and ordinary differential equations (ODEs) in a software package (PhysioLab Modeler®, Entelos Holding Corp.). The effects of circulating lipid levels, external sources of plaque inflammation, and inflammatory cell trafficking within the vessel intima are integrated together by the atherosclerosis submodel to predict the rate of progression and/or regression of a “typical” atherosclerotic plaque in a representative vessel, as summarized in Figure 1.

Assumptions and Formulation
The representation for VLDL/IDL penetration, modification, and retention is provided in Figure 2 as an example of modeled physiology, function and relationships. In humans, the major circulating lipid contributing to atherosclerosis is LDL. By contrast, the Apoe-/- mouse primarily possesses TG-rich lipoproteins (IDL and VLDL), but only a minor amount of LDL. The model representation follows the fate of VLDL and IDL particles in the circulation as they enter the vessel wall and are retained in the intima. Influx of lipoprotein particles from the circulation is proportional to the concentration difference of particles in the circulation versus plaque. The rate of influx depends on the area of plaque in contact with the circulation, accounted for by “plaque width” in the 2-dimensional plaque representation. Lipoprotein particles that enter the plaque can undergo further modification and aggregation. Free VLDL/IDL, modified VLDL/IDL, and aggregated VLDL/IDL are represented as distinct states in the platform. Each of these states can undergo enzymatic degradation and then add to the extracellular pool of plaque cholesterol. The lipoprotein modification rate is dependent on inflammation; whereas the rates of aggregation and enzymatic degradation are assumed to be constant. These rates have been calibrated to be consistent with system-level outputs and measurements such as the extracellular concentration of plaque cholesterol and the rates of total plaque cholesterol accumulation.
Parameterization
Parameter values were derived directly from (or calculated to be in agreement with) published data. Preference was given to Apoe-/- mouse data. If unavailable, data from other mouse strains, other animal species, or human cells were used. The implementation of vessel remodelling is a relevant illustration of data usage. Vessel remodelling is the physiological process that occurs when the diameter of the blood vessel increases in an attempt to maintain adequate blood flow through the lumen as a result of changes in turbulence and/or pressure on the vessel wall related to plaque growth [21, 22]. An analysis of absolute and vessel fractional plaque areas revealed a strong linear relationship between plaque area and vessel area in the brachiocephalic artery (BCA) of Apoe-/- mice, Figure 3a. Outward vessel remodelling was therefore implemented by having the vessel area grow as a linear function of plaque area. The slope and y-intercept of this relationship are drawn from a least-squares linear fit of the data for male or female mice. Thus alternate parameterizations may be used depending on gender. Selected parameters were varied across a range consistent with public literature (47 pathways grouped into 21 sets, see Supplemental Table 2) to create individual virtual mice with a diverse set of physiological behaviors. These pathways were selected based on current understanding of the physiology and informed by a sensitivity analysis of the platform (not shown). The resulting cohort encompasses uncertainty in the represented physiology consistent with pre-identified behaviors specific for Apoe-/- mice (see Supplemental Table 3) for internal validation.
Model metrics
Model metrics are summarized in Table 2. To evaluate the representation of particular aspects of the biology (e.g., mathematical functional forms, parameters, associated references), researchers are directed to the full model which contains documentation on the design rationale, use of published data, assumptions, exclusions, and modelling considerations.

Internal validation
The objective of internal validation was to verify that simulations using a single set of selected parameter values (i.e., a single virtual mouse) can reproduce behaviors observed in the literature. Virtual mice were considered internally validated if their simulated plaque progression was within the range reported in response to a range of stimuli such as chow diet, high fat diet, and ezetimibe treatment (see Supplemental Table 3). Moreover, simulated plaque progression rates for the cohort were consistent with observations in key Apoe-/- double knockout (PGI2, TXA2, and superoxide) studies [23, 24]. Validated virtual mice exhibited also cholesterol profiles consistent with those observed experimentally in our laboratories.
Validation by comparing model and in-vivo data
As cigarette smoke exposure is a known risk factor of atherosclerosis, we exposed Apoe-/- mice to the Reference Cigarette 3R4F to test model-generated predictions of plaque progression upon smoking and cessation


Experimental study design
All animal experimental procedures were performed at Philip Morris Research Laboratories Belgium and were approved by the Institutional Animal Care and Use Committee (IACUC). Female Apoe-/- mice (Taconic) aged 8 to 10 weeks were randomly allocated to groups of 15 animals and were fed a normal chow diet containing 0.003% cholesterol and 4.5% fat (Harlan Teklad 2014, Harlan, Oxon UK). Mice were exposed to mainstream smoke (MS) of the 3R4F Reference Cigarette at a concentration of 600 mg total particulate matter (TPM)/m3 or to filtered, conditioned fresh air (sham) for 3 or 4 hours/day, 5 days/week for up to 6 months, or were switched after 3 months of MS exposure to sham exposure for additional 3 months (“cessation group”). Smoke from the 3R4F Reference Cigarette (University of Kentucky, Lexington, KY, USA) [25] was generated on a modified automatic 30-port smoking machine (INBIFO type SM85i) [26]. The cigarettes were conditioned and smoked in basic accordance with the International Organization for Standardization (ISO) standards 3402 (ISO, 1999) and 3308 (ISO, 2000). Data derived from the first 4 months of treatment (3 months of exposure + 1 month of either exposure or cessation) was used to provide input to the model and later time points serving as the basis for evaluating the predictions (validation study). A similar study was conducted earlier to provide calibration data points (calibration study).
Determination of lipoprotein profiles
Serum was analyzed for total cholesterol using a commercially available kit (Thermo Clinical Labsystems, Frankfurt, Germany) according to manufacturer’s instructions. VLDL, LDL/IDL, and HDL were separated by high performance liquid chromatography (HPLC) and measured photometrically. Total cholesterol was calculated as the sum of the peak areas for VLDL, LDL/IDL, and HDL.
Determination of arachidonic acid metabolites
Selected isoprostane metabolites (8-iso PGF2a, 2,3 dinor- 8-iso PGF2a, 6-keto PGF1a, 2,3 dinor-6-keto PGF 1a) and thromboxane metabolites (11dhTXB2 , 2,3 dinorTXB2 , 2,3 dinorTXB2) were simultaneously quantitatively analyzed in aliquots of overnight collected urine using LC-MS/MS.
Calculation of atherosclerotic plaque area
BCA samples were fixed in 4% formalin and embedded in paraffin. 5 µm cross sections of the BCA were mounted and stained with hematoxylin-eosin starting after the branch of the arteria subclavia dextra and every 100 µm thereafter. Plaque area evaluation in the BCA was performed as described previously [27] with the difference that at least 4 cross-sections were averaged per mouse. The aortic arch was sliced longitudinally, flattened, and fixed with pins. DISKUS® image analysis software (Hilgers, Koenigswinter, Germany) was used to calculate plaque area. The intimal area covered by plaques was determined and normalized to the whole aortic arch area [28].
Simulation of conventional cigarette smoke exposure
To simulate the impact of conventional cigarette smoke exposure in the model, pathways known to be affected were simultaneously modulated consistent with our laboratory experience and/or with public reports (see Supplemental Table 4) resulting in multiple alternate hypotheses (n=105) for the effect of 3R4F exposure (see Supplemental Figure 1). Each hypothesis was calibrated such that the virtual mouse cohort matched an experimental calibration dataset for changes in both cholesterol profiles and plaque area in response to 3R4F exposure (see Supplemental Table 5).
Simulation of cessation
Experimental data demonstrated the rapid return of lipoprotein levels to baseline as early as one month after cessation (see Supplemental Figure 2). Thus cessation in the model was implemented as an instantaneous removal of the smoke exposure effect on lipoproteins and extended to the other pathways affected by smoke exposure where no data was available.
Calculation of simulated plaque area
BCA plaque area is calculated as a function of the lipid core cross-sectional area and plaque cap and shoulder volume, which are explicitly represented, Figure 4a. By contrast, aortic arch (AoA) plaque area is computed as a function of BCA plaque area as indicated by a linear relationship observed in the laboratory from the calibration dataset. The BCA/AoA relationship is dependent on experimental conditions (e.g., smoke exposure), Figure 4b, and thus the appropriate relationship was applied to make predictions for AoA under sham or 3R4F exposure. For cessation, the sham relationship was used to be consistent with the observation that cessation normalizes cholesterol levels, see Supplemental Figure 2.
Results
Virtual mouse cohort characteristics
Twenty-three cholesterol profiles (combinations of VLDL, IDL/LDL, and HDL) consistent with experimental data were represented, Figure 5a. From these cholesterol profiles, over 20,000 virtual mice were created by varying parameters as indicated in Supplementary Table 2. Clustering was conducted with the parameter values that vary between virtual mice to limit mechanistic redundancy in the cohort. The final cohort (n=1644) spanned the range of values explored for each parameter, Figure 5b, and thus encompasses mechanistic diversity that may explain experimental variability. In support of this, the distribution of BCA plaque area and total cholesterol level in the chow-fed virtual cohort were consistent with the observed experimental variability for chow-fed Apoe-/- mice, Figure 5c (left). These experimental data also clearly demonstrate that there are variations in baseline plaque progression rates in Apoe-/- mice that must be considered in simulations as a wide range of BCA plaque areas can be observed over a similar range of cholesterol level. Therefore, we assigned prevalence weights to individual mice such that the cohort matched the mean and 95% confidence interval of the 3 month experimental total cholesterol data from a calibration dataset while considering three scenarios of baseline plaque progression, Figure 5c (right). Lastly, upon simulated reductions in PGI2, TXA2, and superoxide, the virtual mouse cohort exhibited responses for plaque progression consistent with experimental data [23, 24], thereby providing further confidence in the modeled physiology as these interventions are mechanistically distinct, Figure 5d.

Predictions for plaque progression
The Apoe-/- Mouse PhysioLab platform was used to predict mean and standard deviation for BCA and AoA plaque area at 6 months for sham, 3R4F exposure, and cessation (3 months 3R4F exposure followed by 3 months cessation) using up to 4 month data for impact of smoke exposure on cholesterol profiles and 11dhTXB2 as input. To evaluate the predictions, graphical comparisons were made between in vivo data of the validation study and simulation results. An estimate of the uncertainty associated with the experimental data was assessed by bootstrapping (with replacement) due to the small sample size in each experimental arm (8-16 animals per arm). Each data set was bootstrapped 10,000 times. The 95% confidence intervals for the sample means and SDs were computed from the results of the bootstrapping. Simulations of the virtual mouse cohort (n=1644) exposed to each of the 105 3R4F hypotheses were evaluated under three scenarios for rates of baseline plaque progression, generating 105 x 3 = 315 forecasts per virtual mouse. The forecasts were plotted on the same axis as the bootstrapping results to visually demonstrate the degree of overlap.
Initially, the bootstrap was performed only with data from the validation experiments (see Supplemental Table 6). It was clear, however, that there were significant differences between the calibration and validation datasets. Comparison of bootstrap results indicated notable differences in mean AoA plaque areas (see Supplemental Figure 3). This result likely reflects the inherent variability in in vivo experiments despite best efforts to control experimental conditions. Consequently, the bootstrap was modified to include both sets of data. Simulation results for both BCA and AoA were in agreement with the bootstrapped experimental data as shown in Figure 6A and Figure 7A, respectively, and as indicated by the significant degree of overlap summarized in Table 3.
Discussion
Atherosclerosis is an ideal disease to apply modelling and simulation approaches as disease progression takes decades before manifestation in clinical events. Thus, any decisions informed by modelling and simulation can save time and hence the financial expense of clinical trials. Many aspects of the disease have been quantitatively investigated, which allows for application of various modelling approaches. For example, several groups have reported computational models that focus

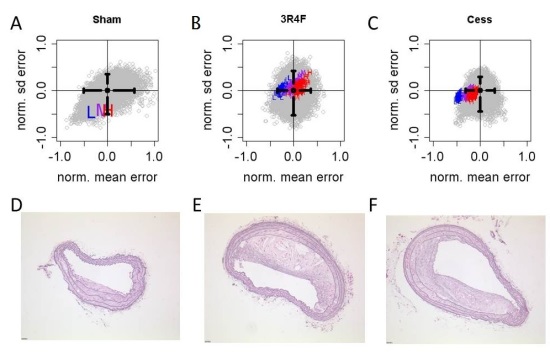
on hemodynamics and its relationship to atherosclerosis [29-31]. Some of these models have been customized with individual patient data [32, 33]. Other models have incorporated the role of inflammation [34, 35]. Plaque progression has also been investigated using agent-based [36] and ODE approaches [37]. While these approaches have yielded insights into individual aspects of the disease process, they fall short of making population-level predictions and their clinical utility is unclear. We employed an ODE-based, top-down, approach to successfully predict plaque progression in the Apoe-/- mouse. This approach has been successfully applied previously to predict outcomes for the NOD mouse in Type 1 diabetes [38, 39].
The Apoe-/- PhysioLab platform was validated by using up to 4 month cholesterol and arachidonic acid data to predict 6 month plaque area endpoints for the impact of 3R4F exposure and cessation. The Apoe-/- PhysioLab accurately forecasted plaque area progression observed experimentally in sham mice, as well as mice exposed to 3R4F, and those that were subject to a cessation protocol. Accordingly, the Apoe-/- PhysioLab may be used by researchers to investigate the impact of other interventions and predict dynamic changes in plaque progression. Dosing schedules could thus be optimized in silico to increase the efficiency and reduce the cost of preclinical laboratory experiments.
The validated Apoe-/- PhysioLab also provides proof-of-concept for modelling this complex disease, suggesting that a similar approach can be successful for the human scenario [40], where experimental validation data is much more difficult to obtain. In addition to predicting mean responses, the Apoe-/- PhysioLab was also able to predict standard deviations by accounting for several sources of uncertainty: 1) variability observed in the reported data for underlying mechanistic pathways; and 2) variability in the reported data for the effect of 3R4F exposure on individual pathways. These uncertainties are managed by the Apoe-/- PhysioLab as alternate parameterizations represented by different virtual mice and alternate hypotheses for 3R4F exposure, respectively.


We present a model capable of predicting plaque outcomes in the Apoe-/- mouse. Ultimately, we envision using the Apoe-/- PhysioLab platform as a translational bridge to the human model [40] by enabling direct translation of changes in specific biomarkers and mediators in pre-clinical Apoe-/- mouse studies to appropriately-scaled responses in the human, where the impact on CHD risk could be investigated. In order to accomplish this, the next generation Apoe-/- PhysioLab platform may need to consider how plaque stability is related between mice and humans. Indeed, a recent model has been described using histology-based finite element analysis to explain the stability of aortic lesions in Apoe-/- mice relative to BCA lesions [41], which have been reported to undergo cap rupture [42].
A fully integrated modelling and simulation approach that considers the biological variability inherent in the human population could yield candidate biomarkers that may predict both plaque progression and CHD risk by mining simulation data in virtual patients whose parameterizations represent known genetic and physiological variability in humans. While such an approach still requires empirical validation, it has advantages over other data mining techniques in that it uses a broader set of input data from which to propose candidate markers. In support of this, the top-down approach has been successfully used in rheumatoid arthritis to propose markers that predict disease progression [43, 44].
Another potential use of the modelling approach detailed in this manuscript is the ability to conduct sensitivity analyses to identify key pathways that are required for clinical response. Such information may be used to guide experiments to identify or prioritize lead therapeutic candidates. Additionally, these analyses may also reveal novel therapeutic targets and/or combinations of targets that may be more clinically effective. In conclusion, the Apoe-/- PhysioLab platform lays the foundation for the use of modelling and simulation in the study of atherosclerosis. The top-down modelling approach holds promise for shaping the development of novel therapeutics and interventions for this widespread disease.
Acknowledgements
We gratefully acknowledge Chris Brunell, James Herro, Mark Zhang, and Brian Schmidt for software and database support. We also acknowledge Walter Schlage, Rosemarie Lichtner, Christelle Haziza, and Gaëlle Diserens for their valuable support throughout the collaboration.
References
- Lloyd-Jones D, Adams RJ, Brown TM, Carnethon M, Dai S, De Simone G et al. Heart disease and stroke statistics–2010 update: a report from the American Heart Association. Circulation 2010; 121(7): e46-e215.
REFERENCE LINK - Nissen SE, Wolski K. Effect of rosiglitazone on the risk of myocardial infarction and death from cardiovascular causes. New Engl J Med 2007; 356(24): 2457-2471.
REFERENCE LINK - Zhang SH, Reddick RL, Piedrahita JA, Maeda N. Spontaneous hypercholesterolemia and arterial lesions in mice lacking apolipoprotein E. Science 1992; 258(5081): 468-471.
REFERENCE LINK - Coleman R, Hayek T, Keidar S, Aviram M. A mouse model for human atherosclerosis: long-term histopathological study of lesion development in the aortic arch of apolipoprotein E-deficient (E0) mice. Acta Histochem 2006; 108(6): 415-424.
REFERENCE LINK - Ley K, Miller YI, Hedrick CC. Monocyte and macrophage dynamics during atherogenesis. Arterioscl Throm Vas 2011; 31(7): 1506-1516.
REFERENCE LINK - Adamson S, Leitinger N. Phenotypic modulation of macrophages in response to plaque lipids. Curr Opin Lipidol 2011; 22(5): 335-342.
REFERENCE LINK - Williams KJ, Tabas I. Lipoprotein retention–and clues for atheroma regression. Arterioscl Throm Vas 2005; 25(8): 1536-1540.
REFERENCE LINK - Assmann G, Gotto AM. HDL cholesterol and protective factors in atherosclerosis. Circulation 2004; 109(23 Suppl 1): III8-14.
REFERENCE LINK - Llodrá J, Angeli V, Liu J, Trogan E, Fisher EA, Randolph GJ. Emigration of monocyte-derived cells from atherosclerotic lesions characterizes regressive, but not progressive, plaques. P Natl Acad Sci USA 2004; 101(32): 11779-11784.
REFERENCE LINK - Simionescu M. Implications of early structural-functional changes in the endothelium for vascular disease. Arterioscl Throm Vas 2007; 27(2): 266-274.
REFERENCE LINK - Steinmetz M, Nickenig G, Werner N. Endothelial-regenerating cells: an expanding universe. Hypertension 2010; 55(3): 593-599.
REFERENCE LINK - Foteinos G, Hu Y, Xiao Q, Metzler B, Xu Q. Rapid endothelial turnover in atherosclerosis-prone areas coincides with stem cell repair in apolipoprotein E-deficient mice. Circulation 2008; 117(14): 1856-1863.
REFERENCE LINK - Singh U, Jialal I. Oxidative stress and atherosclerosis. Pathophysiology. 2006; 13(3): 129-142.
REFERENCE LINK - Custodis F, Baumhäkel M, Schlimmer N, List F, Gensch C, Böhm M, et al. Heart rate reduction by ivabradine reduces oxidative stress, improves endothelial function, and prevents atherosclerosis in apolipoprotein E-deficient mice. Circulation 2008; 117(18): 2377-2387.
REFERENCE LINK - Sato H, Kato R, Isogai Y, Saka G, Ohtsuki M, Taketomi Y, et al. Analyses of group III secreted phospholipase A2 transgenic mice reveal potential participation of this enzyme in plasma lipoprotein modification, macrophage foam cell formation, and atherosclerosis. J Biol Chem 2008; 283(48): 33483-33497.
REFERENCE LINK - d? Uscio LV, Katusic ZS. Increased vascular biosynthesis of tetrahydrobiopterin in apolipoprotein E-deficient mice. Am J Physiol-Heart C 2006; 290(6): H2466-71.
REFERENCE LINK - Laursen JB, Somers M, Kurz S, McCann L, Warnholtz A, Freeman BA, et al. Endothelial regulation of vasomotion in apoE-deficient mice: implications for interactions between peroxynitrite and tetrahydrobiopterin. Circulation 2001; 103(9): 1282-1288.
REFERENCE LINK - Ozaki M, Kawashima S, Yamashita T, Hirase T, Ohashi Y, Inoue N, et al. Overexpression of endothelial nitric oxide synthase attenuates cardiac hypertrophy induced by chronic isoproterenol infusion. Circ J 2002; 66(9): 851-856.
REFERENCE LINK - Vinh A, Widdop RE, Drummond GR, Gaspari TA. Chronic angiotensin IV treatment reverses endothelial dysfunction in ApoE-deficient mice. Cardiovasc Res 2008; 77(1): 178-187.
REFERENCE LINK - Lievens D, von Hundelshausen P. Platelets in atherosclerosis. Thromb Haemostasis 2011; 106(5): 827-838.
REFERENCE LINK - Bonthu S, Heistad DD, Chappell DA, Lamping KG, Faraci FM. Atherosclerosis, vascular remodeling, and impairment of endothelium-dependent relaxation in genetically altered hyperlipidemic mice. Arterioscl Throm Vas 1997; 17(11): 2333-2340.
REFERENCE LINK - Lutgens E, de Muinck ED, Heeneman S, Daemen MJ. Compensatory enlargement and stenosis develop in apoE(-/-) and apoE*3-Leiden transgenic mice. Arterioscl Throm Vas 2001; 21(8): 1359-1365.
REFERENCE LINK - Barry-Lane PA, Patterson C, van der Merwe M, Hu Z, Holland SM, Yeh ET, et al. p47phox is required for atherosclerotic lesion progression in ApoE(-/-) mice. J Clin Invest 2001; 108(10): 1513-1522.
- Kobayashi T, Tahara Y, Matsumoto M, Iguchi M, Sano H, Murayama T, et al. Roles of thromboxane A(2) and prostacyclin in the development of atherosclerosis in apoE-deficient mice. J Clin Invest 2004; 114(6): 784-794.
- Davies HVA. The Reference Cigarette. Lexington, KY: Kentucky Tobacco Research & Development Center (KTRDC); 2003.
- Haussmann HJ, Anskeit E, Becker D, Kuhl P, Stinn W, Teredesai A, et al. Comparison of fresh and room-aged cigarette sidestream smoke in a subchronic inhalation study on rats. Toxicol Sci 1998; 41(1): 100-116.
REFERENCE LINK - Stolle K, Berges A, Lietz M, Lebrun S, Wallerath T. Cigarette smoke enhances abdominal aortic aneurysm formation in angiotensin II-treated apolipoprotein E-deficient mice. Toxicol Lett 2010; 199(3): 403-409.
REFERENCE LINK - von Holt K, Lebrun S, Stinn W, Conroy L, Wallerath T, Schleef R. Progression of atherosclerosis in the Apo E-/- model: 12-month exposure to cigarette mainstream smoke combined with high-cholesterol/fat diet. Atherosclerosis 2009; 205(1): 135-143.
REFERENCE LINK - Chatziprodromou I, Poulikakos D, Ventikos Y. On the influence of variation in haemodynamic conditions on the generation and growth of cerebral aneurysms and atherogenesis: a computational model. J Biomech 2007; 40(16): 3626-3640.
REFERENCE LINK - Olgac U, Kurtcuoglu V, Poulikakos D. Computational modeling of coupled blood-wall mass transport of LDL: effects of local wall shear stress. Am J Physiol-Heart C 2008; 294(2): H909-19.
REFERENCE LINK - Liu B, Tang D. Computer simulations of atherosclerotic plaque growth in coronary arteries. Mol Cell Biomech 2010; 7(4): 193-202.
- Olgac U, Poulikakos D, Saur SC, Alkadhi H, Kurtcuoglu V. Patient-specific three-dimensional simulation of LDL accumulation in a human left coronary artery in its healthy and atherosclerotic states. Am J Physiol-Heart C 2009; 296(6): H1969-82.
REFERENCE LINK - Sun N, Torii R, Wood NB, Hughes AD, Thom SAM, Xu XY. Computational modeling of LDL and albumin transport in an in vivo CT image-based human right coronary artery. J Biomech Eng 2009; 131(2): 021003.
REFERENCE LINK - Ibragimov AI, McNeal CJ, Ritter LR, Walton JR. A mathematical model of atherogenesis as an inflammatory response. Math Med Biol 2005; 22(4): 305-333.
REFERENCE LINK - El Khatib N, Génieys S, Kazmierczak B, Volpert V. Mathematical modeling of atherosclerosis as an inflammatory disease. Philos T Roy Soc A 2009; 367(1908): 4877-4886.
- Pappalardo F, Musumeci S, Motta S. Modeling immune system control of atherogenesis. Bioinformatics 2008; 24(15): 1715-1721.
REFERENCE LINK - Ougrinovskaia A, Thompson RS, Myerscough MR. An ODE model of early stages of atherosclerosis: mechanisms of the inflammatory response. B Math Biol 2010; 72(6): 1534-1561.
REFERENCE LINK - Shoda L, Kreuwel H, Gadkar K, Zheng Y, Whiting C, Atkinson M, et al. The Type 1 Diabetes PhysioLab Platform: a validated physiologically based mathematical model of pathogenesis in the non-obese diabetic mouse. Clin Exp Immunol 2010; 161(2): 250-267.
- Fousteri G, Chan JR, Zheng Y, Whiting C, Dave A, Bresson D, et al. Virtual optimization of nasal insulin therapy predicts immunization frequency to be crucial for diabetes protection. Diabetes 2010; 59(12): 3148-3158.
REFERENCE LINK - Powell, Lyn; Lo, Arthur; Cole, MS; Trimmer J. Application of predictive biosimulation to the study of atherosclerosis: development of the cardiovascular PhysioLab platform and evaluation of CETP inhibitor therapy. Proceedings of the FOSBE 2007.
- Vengrenyuk Y, Kaplan TJ, Cardoso L, Randolph GJ, Weinbaum S. Computational stress analysis of atherosclerotic plaques in ApoE knockout mice. Ann Biomed Eng 2010; 38(3): 738-747.
REFERENCE LINK - Williams H, Johnson JL, Carson KGS, Jackson CL. Characteristics of intact and ruptured atherosclerotic plaques in brachiocephalic arteries of apolipoprotein E knockout mice. Arterioscl Throm Vas 2002; 22(5): 788-792.
REFERENCE LINK - Meeuwisse CM, van der Linden MP, Rullmann TA, Allaart CF, Nelissen R, Huizinga TW, et al. Identification of CXCL13 as a marker for rheumatoid arthritis outcome using an in silico model of the rheumatic joint. Arthritis Rheum 2011 May; 63(5): 1265-1273.
REFERENCE LINK - Rosengren S, Kalunian KC, Kavanaugh A, Boyle DL. CXCL13 as a marker for outcome of rheumatoid arthritis: Comment on the article by Meeuwisse et al. Arthritis Rheum 2011; 63(11): 3646-3647.
REFERENCE LINK
ces”>